Search and visualization of polysaccharides containing target monosaccharides from glycan databases.
Summary
My name is Ryu Takayanagi, a second-year master's student at the University of Tokyo, currently interning at digzyme. At university, I have been conducting research related to protein phosphorylation and protein tertiary structures.
In this tech blog, I would like to introduce GlycoSearcher, a new tool we have developed as part of our R&D activities for comprehensive search and visualization of polysaccharides containing target monosaccharides.
In recent years, research and industrial utilization of polysaccharides, such as starch and dietary fiber, have become increasingly active. There is a growing demand for the development of new saccharides, and polysaccharides, in particular, are gaining attention for their high structural diversity. To meet this need, we have developed GlycoSearcher, a tool for comprehensive search of various polysaccharides.
Description formats and databases of polysaccharides
The glyco-compounds we are focusing on have already been reported in numbers exceeding hundreds of thousands and have been databased. To selectively identify polysaccharides that fit specific purposes and apply them in fields such as synthesis pathway exploration and enzyme development, a description format that facilitates computational processing and a comprehensive database are essential.
Various methods are known for describing the structure of glyco-compounds (Figure 1). Formats like SNFG and KCF excel in visualization but are not well-suited for advanced computational processing, such as structural information extraction and comparison. On the other hand, the IUPAC format offers a concise structural representation that is readable by both humans and machines, but it struggles with complex and ambiguous expressions, such as repeating units[1]. Therefore, GlycoSearcher employs the WURCS format, which is well-suited for computational processing and can represent repeating units, along with the GlyTouCan database[2], which collects glyco-compound information in the WURCS format.
(Figure 1)
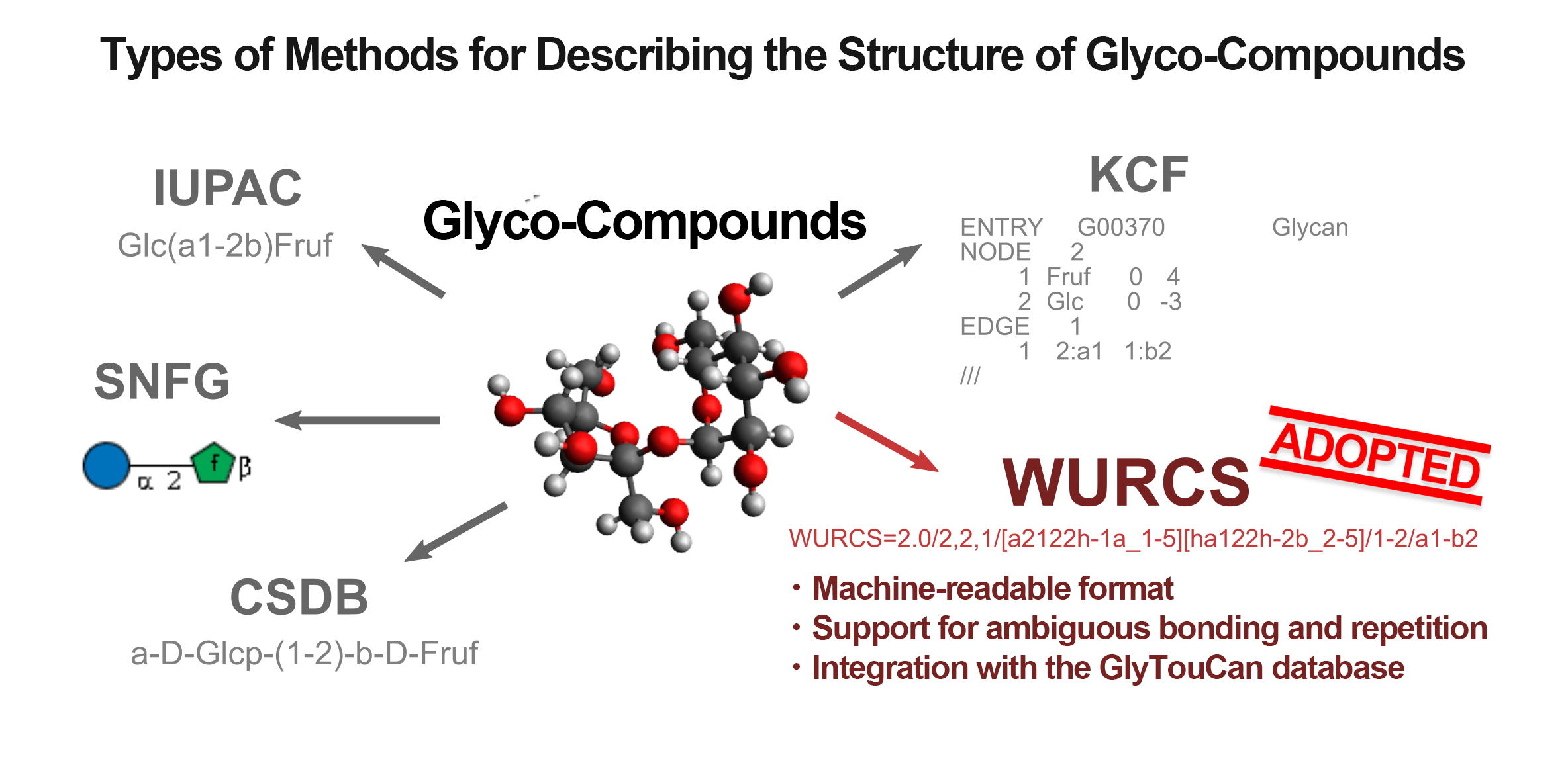
Search Using GlycoSearcher
With GlycoSearcher, it is possible to extract polysaccharides that match specific criteria from a vast number of candidates. For example, you can search for polysaccharides containing particular monosaccharide units such as glucose or galactose. Additionally, it includes a filtering function that allows you to limit the monosaccharide units that make up the polysaccharides. This enables you to list polysaccharides that can be synthesized using a specific monosaccharide as a starting material and other selected sugars.
The results of a search for polysaccharides containing α-glucose are shown below (Figure 2). Out of 219,857 glycan structures, 9,862 polysaccharides containing α-glucose were identified. Further narrowing down the search to polysaccharides consisting only of glucose, galactose, and fructose reduced the number of candidates to 924.
(Figure 2)
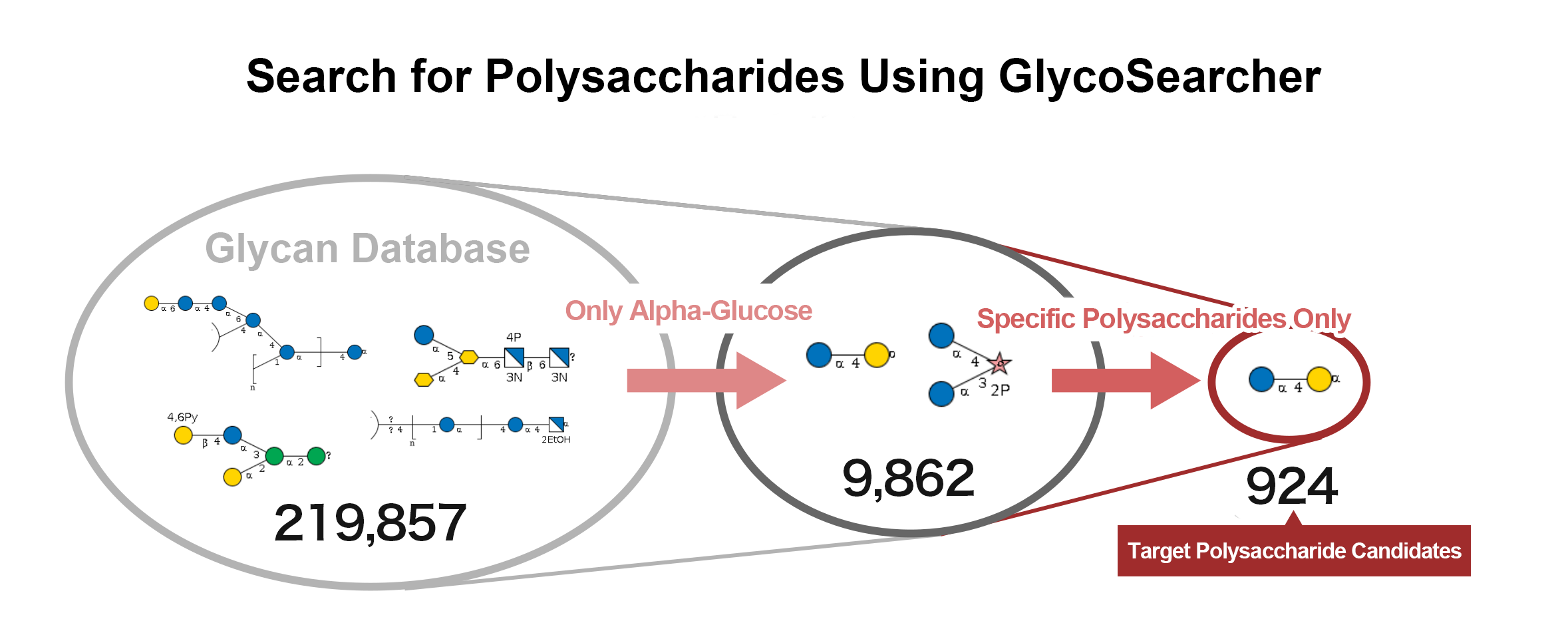
Visualization and feature extraction of polysaccharide structures
The obtained search results can be effectively visualized and utilized for subsequent applications (Figure 3). By reconstructing polysaccharides in WURCS format as graphs, it is possible to rapidly visualize thousands of search results within minutes. Additionally, for structures with ambiguous repeating units, repeating only a specific number of times allows not only the visualization of the actual structure but also facilitates further computational processing, such as structural comparisons that are challenging when ambiguous.
Since the polysaccharides in the search results are represented as graphs, feature extraction for polysaccharide structures is also possible. For example, computations can determine whether the obtained polysaccharide structures have glucose units at their termini or include specific structures (motifs). Furthermore, by integrating the hit polysaccharides with various databases such as PubChem[3], it is possible to obtain information on their common names and related enzyme information, thus providing insights into reactions involving the polysaccharides.
(Figure 3)
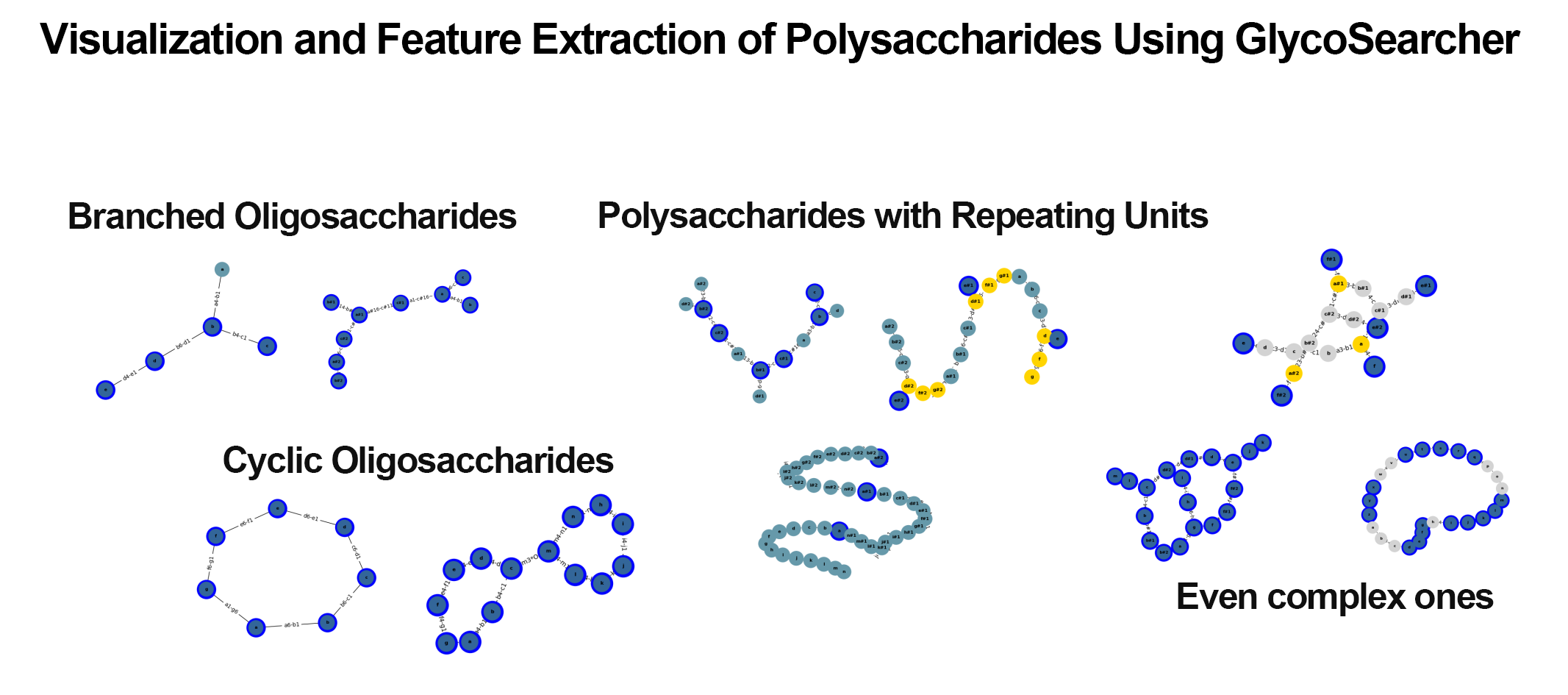
Conclusion
The GlycoSearcher we developed allows for comprehensive searching of target polysaccharides from the database and facilitates further computational processing. Additionally, by extracting information from the identified target polysaccharide candidates and obtaining enzyme information predicted to be involved in their synthesis, we have established a system that links to subsequent enzyme design workflows.
Acknowledgments
The development of GlycoSearcher, including acquiring knowledge about glyco-compounds, was greatly supported by Mr. Isozaki from the Business Development Department. I would like to take this opportunity to express my gratitude.
References
[1] Hosoda, M., & Kinoshita, S. (2021). "Introduction to Glycan-related Informatics." JSBi Bioinformatics Review, 2(1), 87-95.
[2] GlyTouCan. Retrieved from https://glytoucan.org/
[3] PubChem. Retrieved from https://pubchem.ncbi.nlm.nih.gov/
List of answers to the questions we received at our booth at ifia JAPAN 2024 venue.
Our company exhibited at "ifia JAPAN 2024 - The 29th International Food Ingredients/Additives Exhibition & Conference" (organized by Food Chemical News Co., Ltd.), held at Tokyo Big Sight from May 22nd (Wednesday) to 24th (Friday), 2024.
We would like to express our sincere gratitude to everyone who visited our exhibition booth.
In this article, we will introduce and answer the questions we received from all of you during the exhibition period, focusing on the ones that were particularly frequent. Please stay tuned until the end.
Q: What does your company do?
A: In response to our clients' needs, we conduct new enzyme exploration and enzyme modification. By employing our unique bioinformatics technology, which differs from conventional methods, we facilitate rapid enzyme development. We believe that this innovation accelerator can benefit both enzyme manufacturers and food manufacturers.
Q: Do you have any specific examples?
A: In chemical applications, we have successfully explored new enzymes needed by users and achieved significant improvements in enzyme activity. In food applications, we are currently addressing specific themes requested by multiple clients and actively working on them.
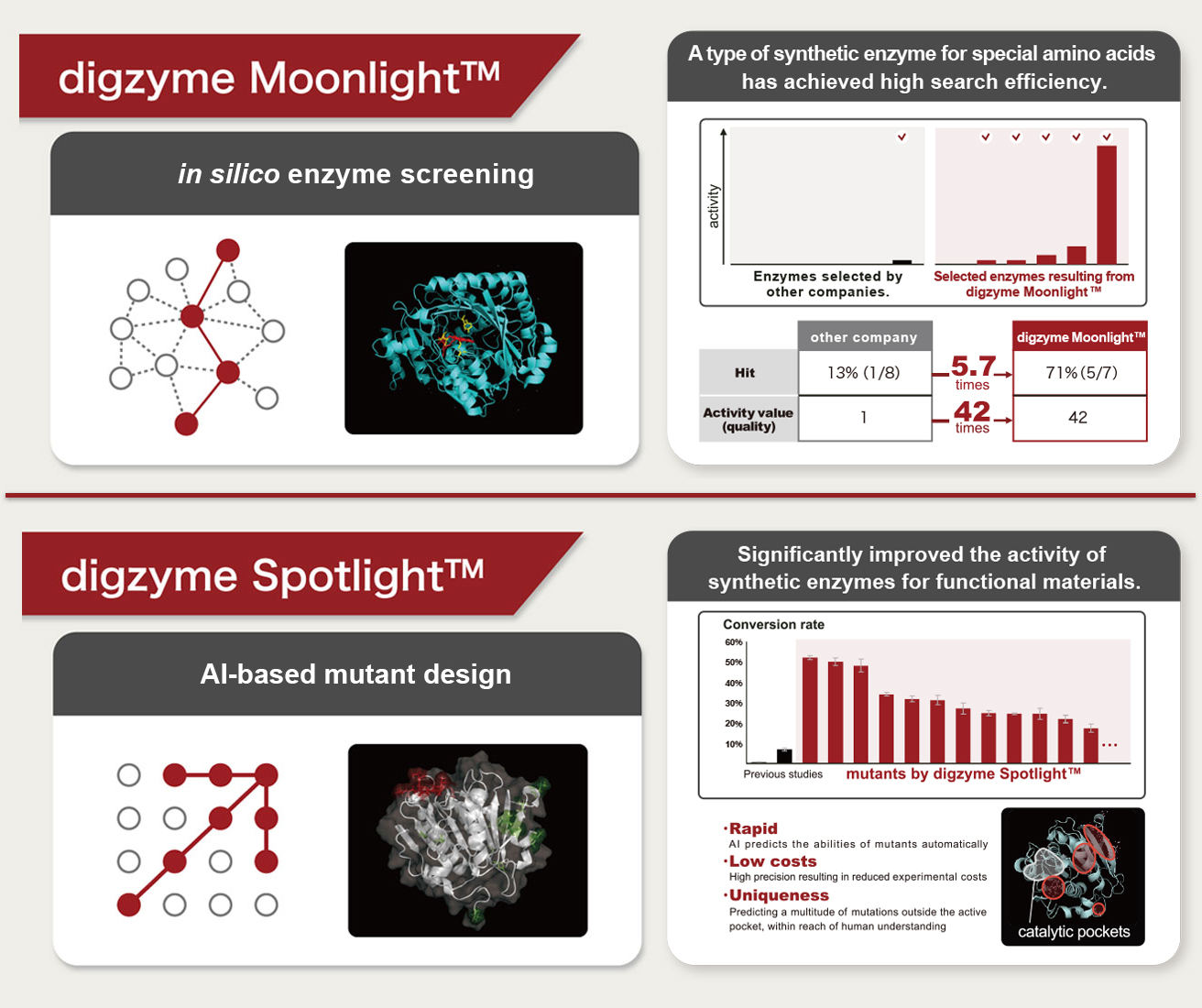
Q: What properties of enzymes can be modified in the digzyme Spotlight (enzyme modification program)?
A: Potential modifications include enhancing activity, improving heat resistance, and altering optimal pH. Modifications to substrate specificity are addressed using the digzyme Moonlight (enzyme exploration program) as needed.
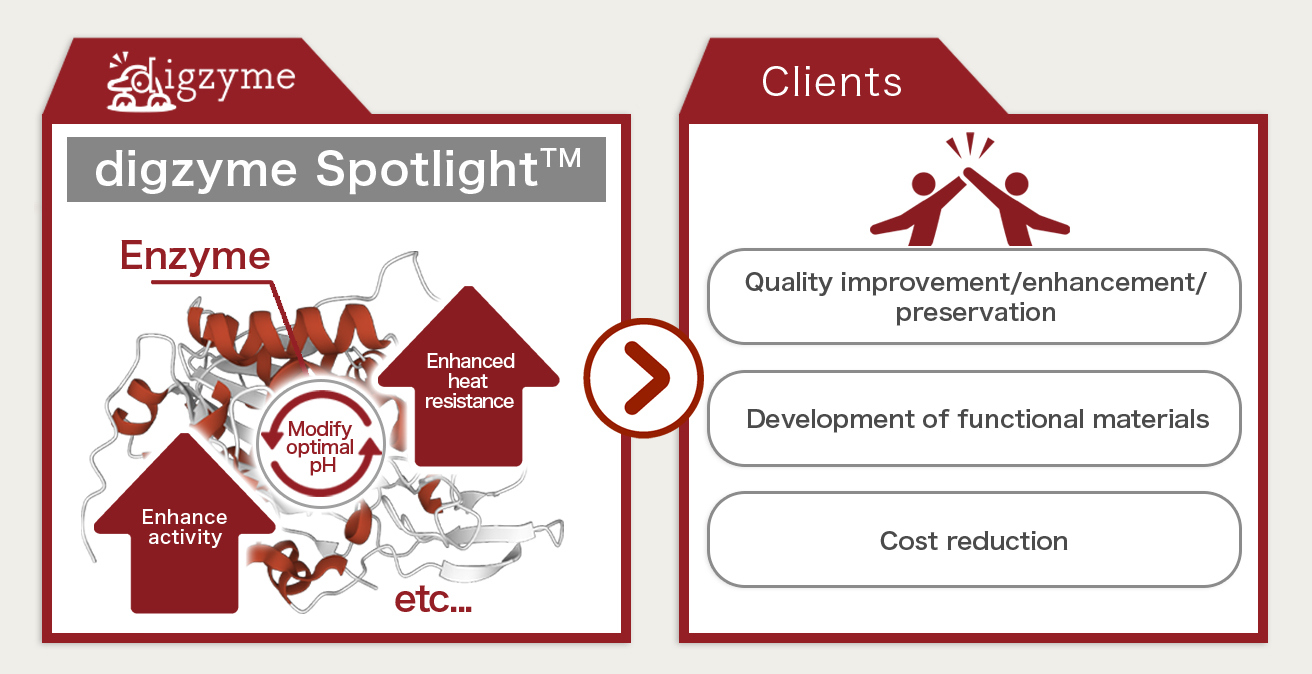
Q: What is the development process like?
A: Depending on the client's situation, we set the start and end goals, but the main process typically follows these steps:
- Development Consultation: We listen to the client's challenges and select the target enzymes.
- Enzyme Design: Using supercomputers, we design the target enzymes.
- Enzyme Library Provision: Enzymes designed on the computer are produced at the lab scale using microorganisms, and the suitability of the enzymes for the intended purpose is verified and confirmed.
- Enzyme Production Provision: We scale up production from the lab to the plant, ensuring stable enzyme supply as a product.
That concludes the Q&A for this article. Thank you very much for reading until the end. If you have any further questions or inquiries, please contact us using the following contact form.
[Contact Form] https://www.digzyme.com/contact/
Compare the predictive accuracy of the machine learning model Spotlight™ for enzyme variants’ activity with prior research.
Summary
Our company offers a service called Spotlight that uses a machine learning model to suggest mutants that improve properties such as enzyme activity and thermostability. We input target enzyme sequences into a pre-trained model using various enzymes to predict mutants with improved activity and thermostability for that enzyme. In this tech blog, we have verified the predictive accuracy of Spotlight™ compared to previous research.
The previous research used for comparison.
In Li et al., 2022, a machine learning model (DLKcat) was created to predict kcat using enzyme amino acid sequences and compounds as input information. To ensure equality in the comparison, we utilized the DLKcat machine learning model algorithm and reconstructed the model using the same training data as Spotlight™, namely the kcat entries from BRENDA. We compared the predicted kcat values of the mutants by the reconstructed DLKcat and Spotlight and evaluated which values were closer to the actual measured values. For this study, we extracted entries from BRENDA, ensuring that only wild type (WT) and single mutant variants were included. Our focus was to compare the sensitivity for a single mutation between the two models.
Results
1. Construction of the machine learning model using BRENDA’s kcat (Turnover Number) data.
Entries for variants with reported kcat values were extracted from BRENDA, including entries for the corresponding wild-type (WT) sequences and information about the compounds used to measure kcat. While ensuring no bias toward specific enzyme families, the entries were divided into a 3:1 ratio of training to test data. Training data consisted of 3,969 entries with an increased kcat, 2,985 entries with an unchanged kcat, and 8,296 entries with a decreased kcat (Figure 1). The test data consisted of 792 entries with an increased kcat, 748 entries with an unchanged kcat, and 1,926 entries with a decreased kcat (Figure 2).
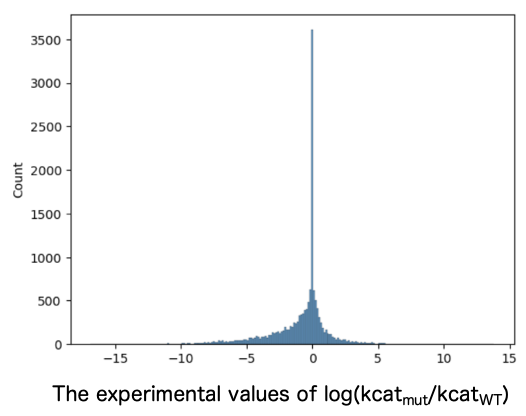
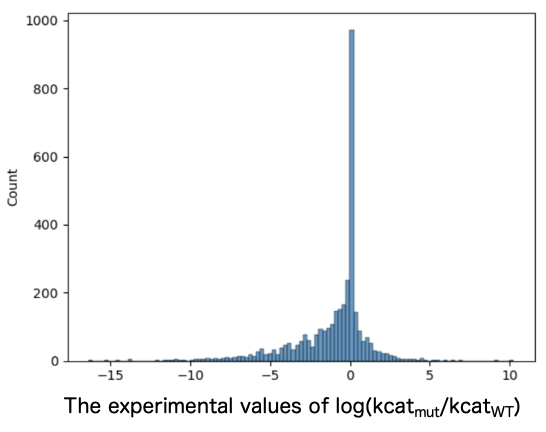
2. Evaluation of mutant/WT ratio of predicted kcat by DLKcat and Spotlight™.
The information from the training data was converted into the format of features required by DLKcat, and a machine learning model was constructed. We converted the training data into the required format of features for Spotlight™ and built a machine learning model (Figure 3).
In the case of DLKcat, the Pearson correlation coefficient between the measured and predicted values of the ratio of the kcat of the mutant to the wild type (WT) kcat was 0.18 (Figure 3). We believe that the reason why the predicted values in DLKcat did not correlate well with the measured values is that DLKcat converts the entire length of the sequence into a vector as a feature, making it difficult for the difference of one amino acid to be reflected in the feature.
In the case of Spotlight™, the Pearson correlation coefficient between the measured and predicted values of the ratio of the kcat of the mutant to the WT kcat was 0.66 (Figure 3). We believe that our Spotlight™ is able to accurately predict the changes caused by single mutations from the WT because it has been devised to reflect the properties of the mutant as a feature.
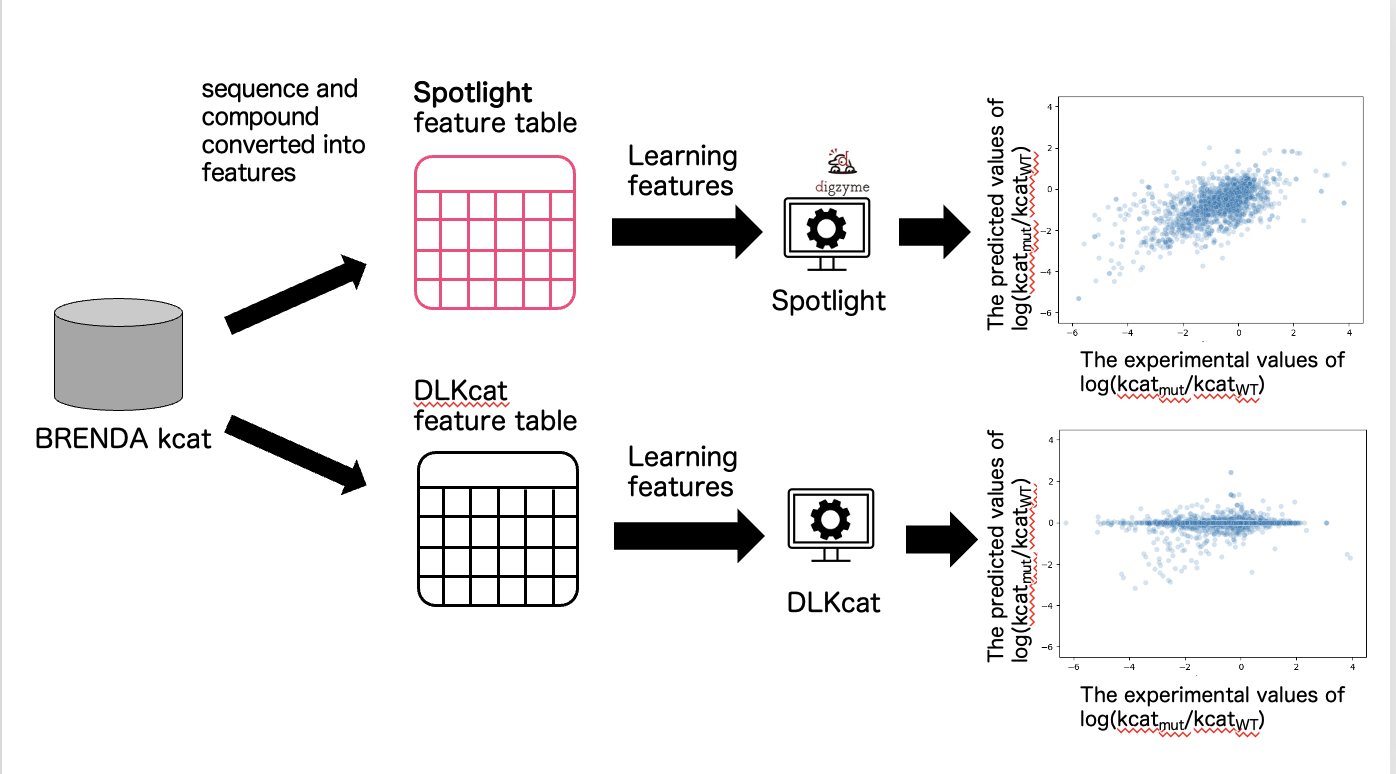
Conclusion
Our Spotlight™ model was found to more accurately predict changes in activity compared to previous research in cases where only one amino acid mutated.
Acknowledgments
We are grateful for the use of data from the following paper to compare the accuracy of enzyme activity prediction in this study.
Li et al., (2022) Deep learning-based kcat prediction enables improved enzyme-constrained model reconstruction. Nature Catalysis.