Practical Example of Enzyme Activity Prediction Using Structure Prediction and MD Simulation
Introduction
I am Isosaki from the Business Development Department. At our company, we are conducting enzyme activity prediction as part of our useful enzyme exploration efforts using molecular dynamics (MD) simulations. From unknown enzyme sequences, we predict their structures and subject the enzyme-ligand complexes to MD simulations. Based on the results, we calculate a proprietary digzyme score to predict enzyme activity. In this blog, we will present an example of predicting the activity of a thiolase-like enzyme, OleA, from its homologous sequences.
Materials Used for Enzyme Activity Prediction
The natural substrate for OleA is acyl-CoA. The enzyme's Cys143 residue cleaves this acyl group. In investigating this activity, a p-nitrophenolate-based experimental system is used (Figure 1).
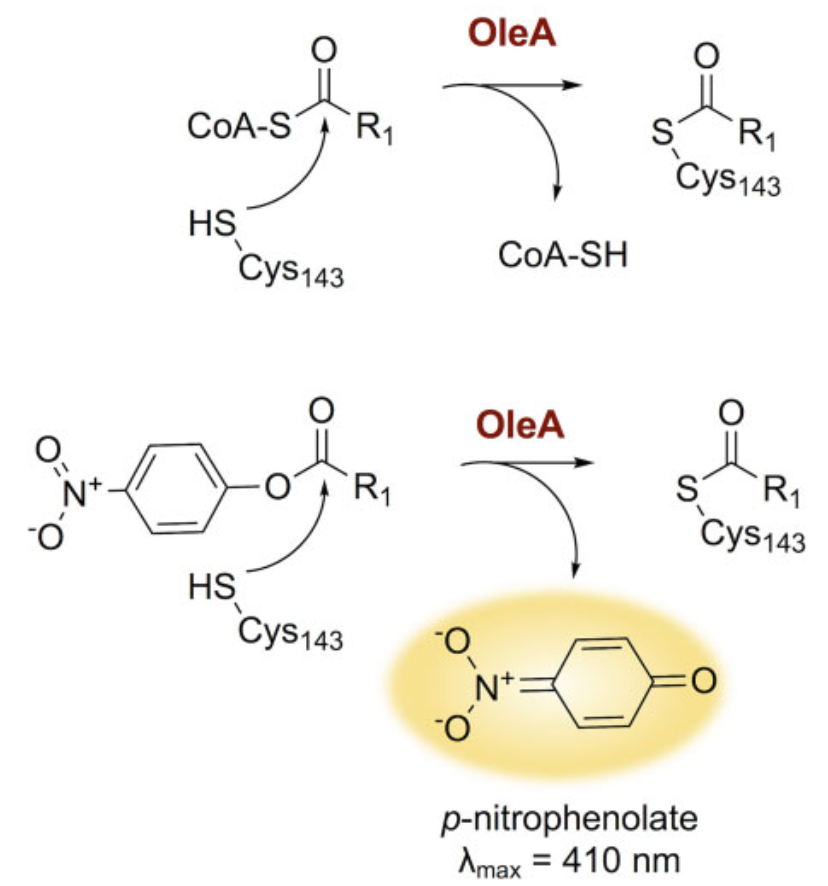
Results
We predicted whether 59 homologous OleA sequences would hydrolyze one type of p-nitrophenolate, specifically 4-nitrophenyl-hexanoate.
1. Prediction of the 3D Structure of 59 Homologous Sequences
First, as all 59 homologous sequences had unknown structures, we predicted their 3D structures. From the predicted structures, we also identified the location of the active residues and the substrate-binding pocket. Figure 2 shows the predicted 3D structure of the homologous sequences and the location of the active residue, Cys. Figure 3 shows the predicted location of the substrate-binding pocket.
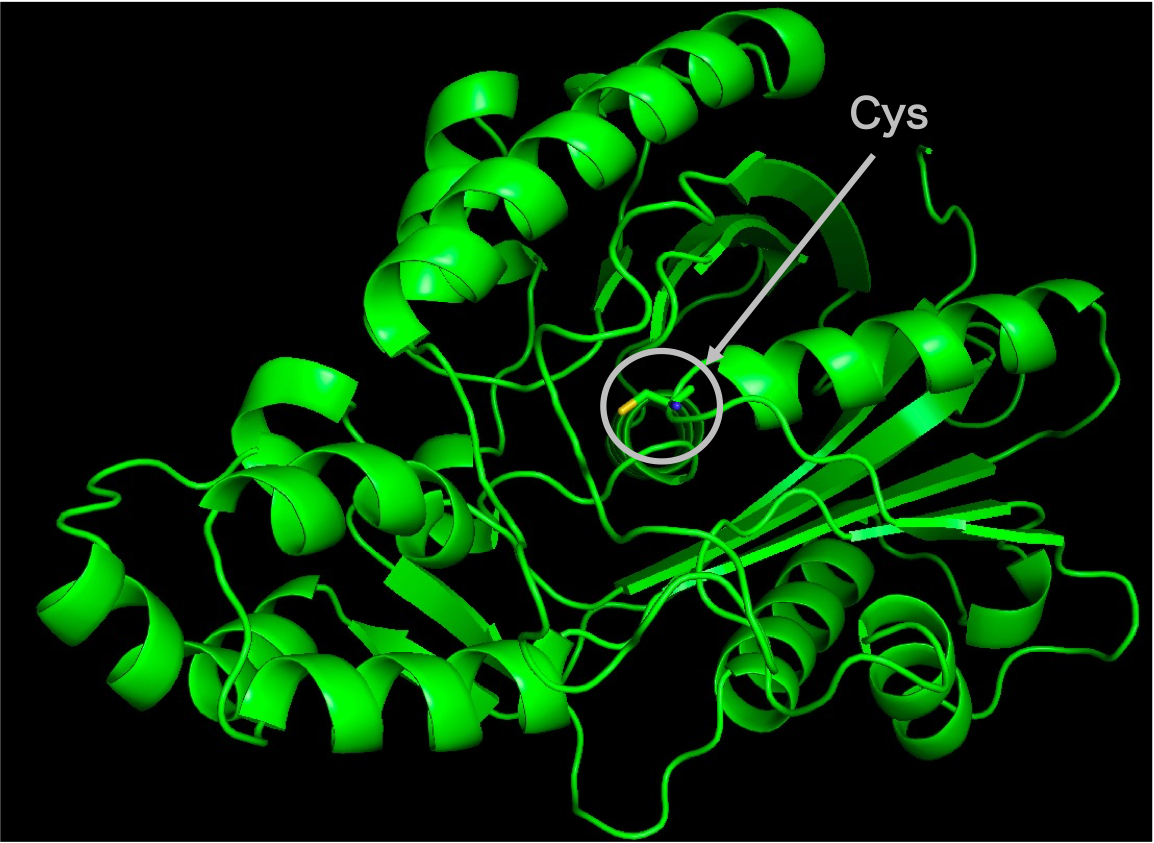
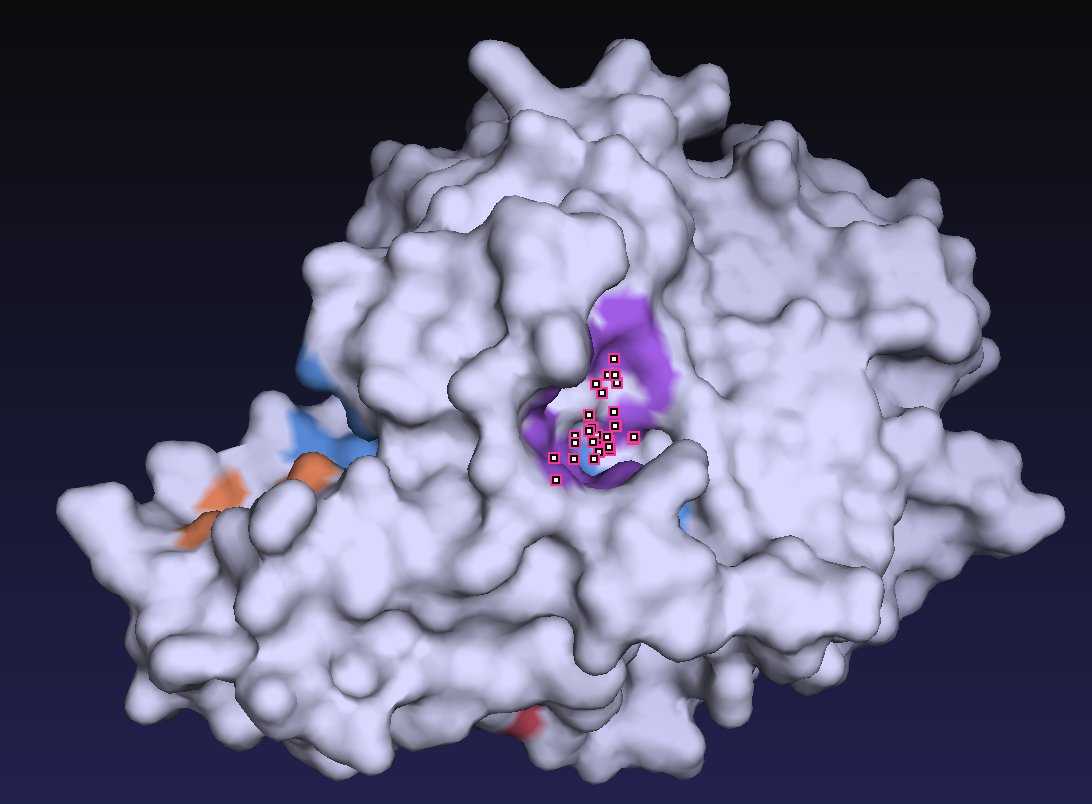
2. Molecular Dynamics Simulation
Next, we placed the enzyme-substrate complex, consisting of the enzyme and 4-nitrophenyl-hexanoate, in a system containing water molecules and ions, and ran molecular dynamics simulations (Figure 4).
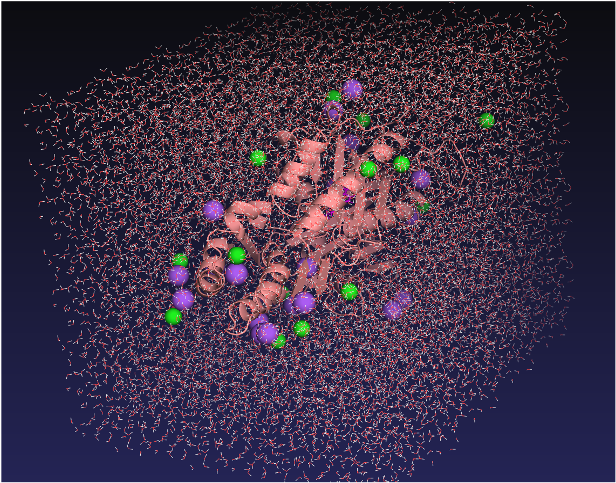
3. Calculation of digzyme's Proprietary Enzyme Activity Prediction Score
Finally, based on the results of the molecular dynamics simulations, we calculate digzyme's proprietary score. Figure 5 shows the predicted scores for all 59 sequences, sorted in descending order. Sequences with confirmed activity from experimental validation are highlighted in pink, while those without activity are shown in gray. Sequences with a score of 70 or higher were classified as active (above the red line in Figure 5). In this case, we predicted 9 sequences to be active, 3 of which were experimentally confirmed to have activity, resulting in a positive predictive value (PPV) of 0.30. The true positive rate (TPR) was 0.6, and the false positive rate (FPR) was 0.13. This indicates that inactive sequences were successfully ranked lower, while active sequences were included in the higher ranks.
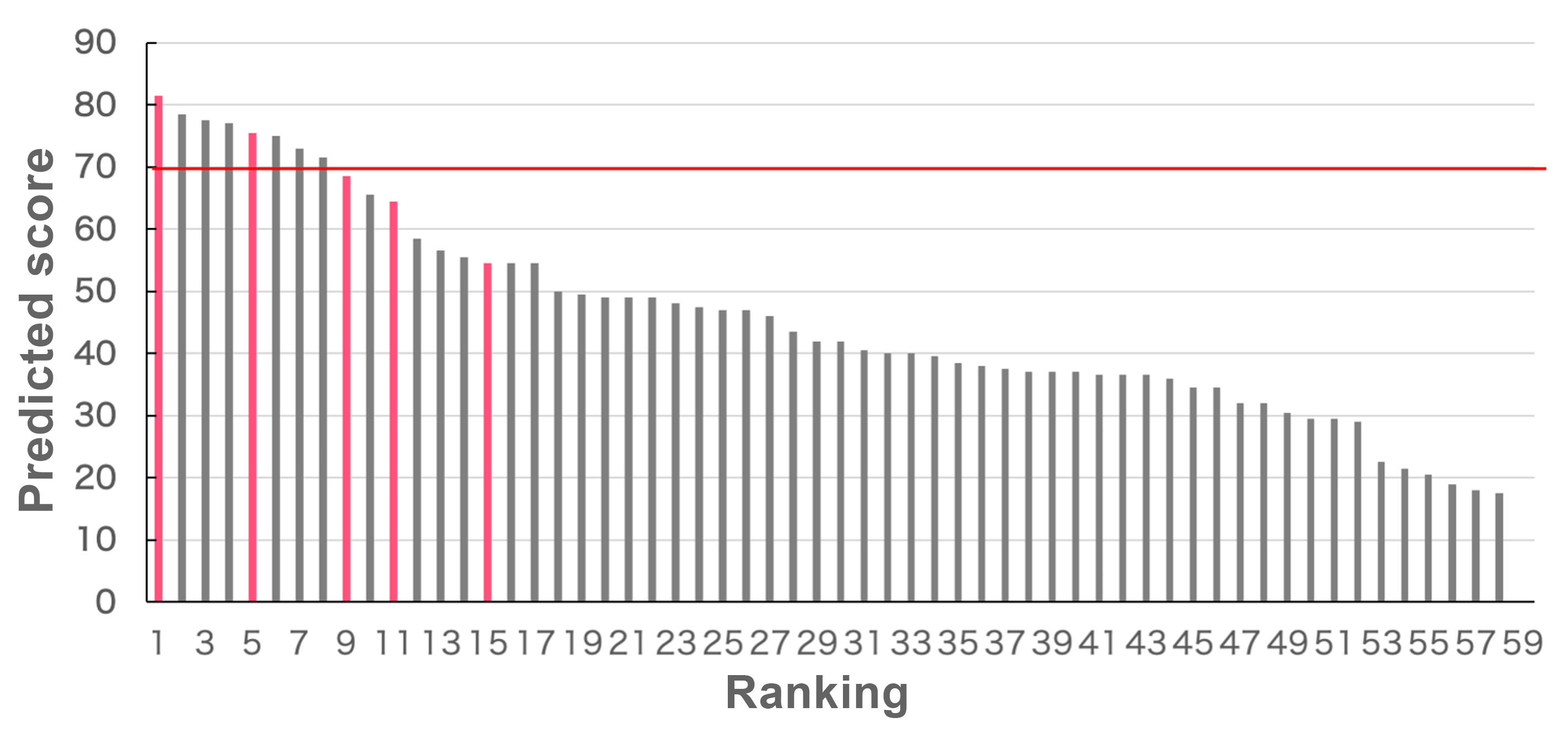
Conclusion
In this blog, we demonstrated the prediction of enzyme activity using our enzyme activity prediction technology, and validated the predictions with experimental results. Typically, 5 to 10 sequences are synthesized for experimental validation. In this case, two of the top five ranked sequences were confirmed to have activity through experiments, demonstrating the practical accuracy of our enzyme activity prediction method. The dataset was specifically selected to simulate a scenario where only a small fraction of the enzyme sequences in the population exhibit activity (in this case, 5 out of 59 sequences). Since the false negative rate was kept low, we successfully predicted enzyme activity with high accuracy.
Acknowledgments
We would like to thank the following paper for providing the experimental data used in this enzyme activity prediction:
Robinson et al., (2020) Machine learning-based prediction of activity and substrate specificity for OleA enzymes in the thiolase superfamily. Synthetic Biology.